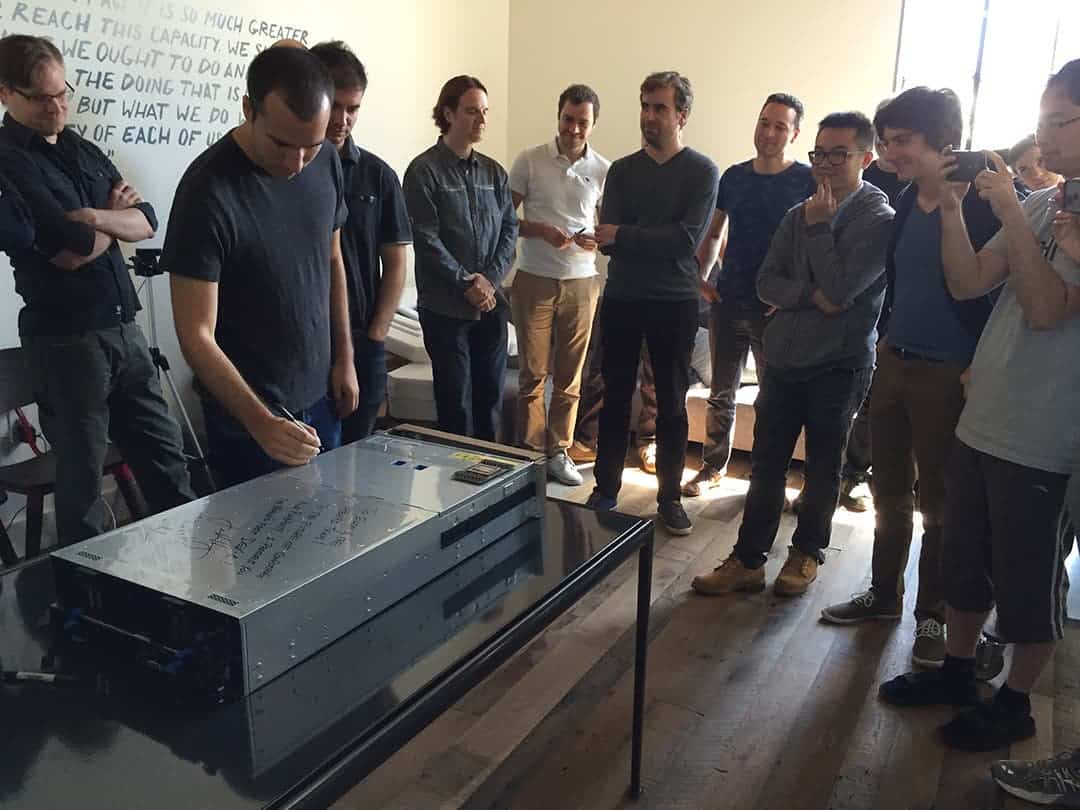
Notes
Fighting the regression
This is a journey through the trials, tactics, and surprises of building a reliable time series model despite relentless regression to the mean.
Read Post
Tokenization
“Tokenization is at the heart of much weirdness of LLMs. Do not brush it off.” AK
Read Post
Applied Ml resource
Thought I'd share this awesome repo for applied ML from the prodigous blogger Eugene Yan :) - https://github.com/eugeneyan/applied-ml
Read Post
Gradient checkpointing
Gradient checkpointing enables you to run a more powerful model on your machine - beneficial under training.
Read Post
Batch processing
A crucial technique in training neural network (NN) models. Instead of processing individual data samples one at a time, batch processing groups multiple samples into batches and processes them simultaneously.
Read Post
Software 2.0
In Software 2.0 most often the source code comprises the dataset that defines the desirable behavior....
Read Post
RAG -> stop hallucinations!
Using them in a RAG architecture brings some different constraints to the table. I think the biggest one is the expectation, especially in a corporate setting, for being factual. But this is not the strength of an LLM. In fact many have deemed the ‘hallucination problem’ a feature and not a bug....
Read Post
Special token injection attacks
A special token is one designed to only be relevant to the model - such as <|end_of_text|>. These can cause standard software engineering bugs if handled poorly in the code.
Read Post
GPU - Model memory
How much GPU do you need? State of the art performance requires state of the art machinery. A100's are not cheap!
Read Post